Insights
Haziqa Sajid
Oct 9, 2024
Learn how AI observability boosts model reliability, reduces errors, and enhances transparency in complex AI systems.
Generative AI is creating new opportunities to boost innovation, streamline operations, and cut costs. McKinsey reports that 65% of companies have already integrated generative AI into their processes. This is double the adoption rate from just a year ago. Experts predict that AI could add up to $4.4 trillion in value to the global economy annually.
However, incorporating AI into operations isn't always simple. The process involves connecting various parts like data pipelines, machine learning models, and computing infrastructure, sometimes leading to unexpected errors.
Discovering the root cause behind model failure can be like finding a needle in a haystack. AI observability is a solution that helps AI engineers find and fix these issues efficiently, all while keeping costs under control.
This blog will discuss how AI observability boosts the reliability of AI systems.
What is AI Observability?
AI observability is an approach to gathering insights on model behavior, performance, and output. It involves tracking key indicators to spot issues like bias, hallucinations, or inaccurate outputs. It also helps ensure that AI systems operate ethically and stay within legal guidelines.
The Growing Need for AI Observability
The need for observability is growing as we integrate AI systems into our decision-making processes. Monitoring AI models ensures transparency, trust, and compliance, especially in high-stakes environments like finance, healthcare, and law.
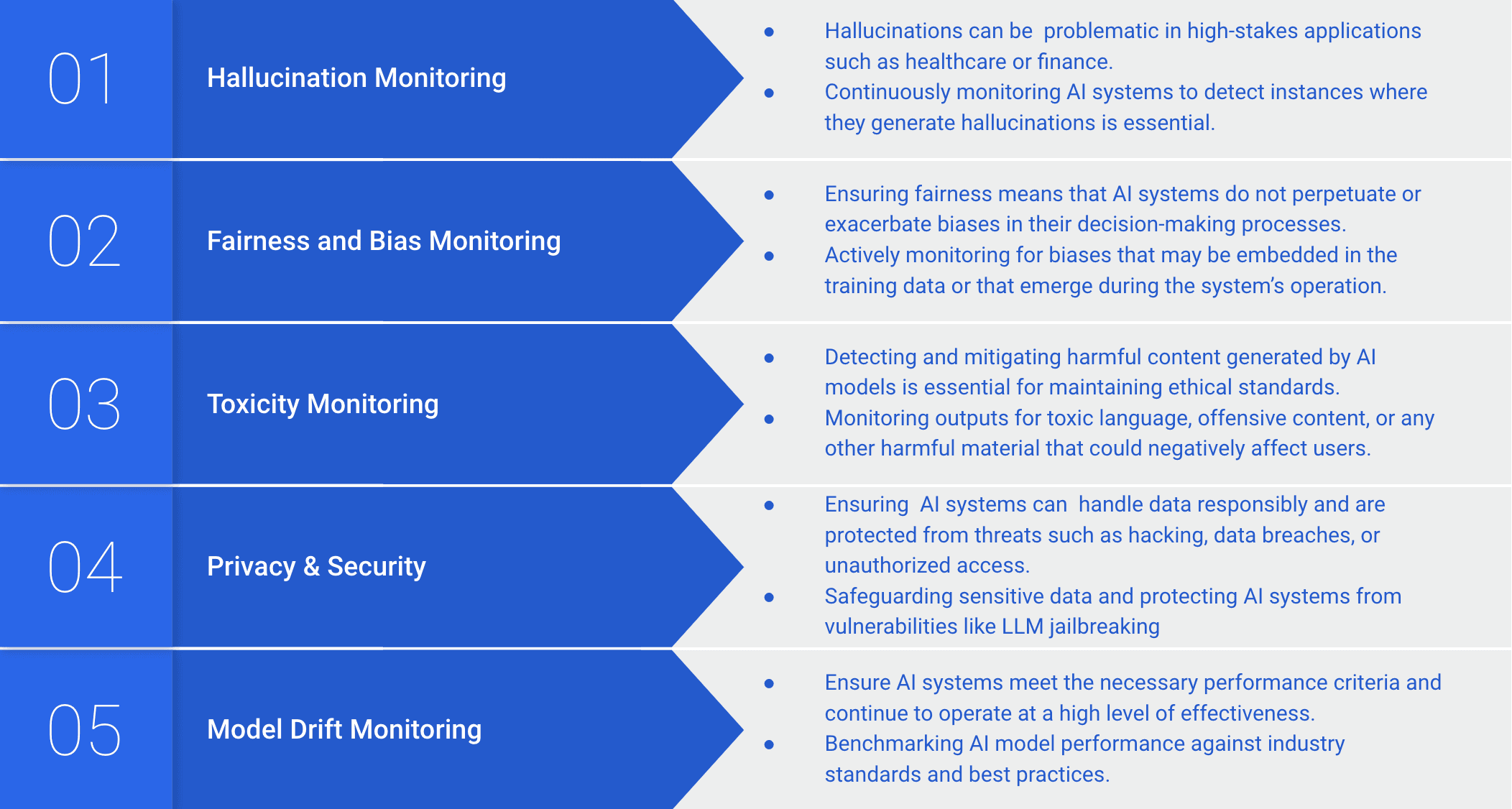
Hallucination Monitoring: AI systems can generate information that appears accurate but isn't grounded in reality. For instance, models like GPT may produce authoritative-sounding but false legal citations, misleading users who might rely on this information for high-stakes decisions.
Fairness and Bias Monitoring: AI systems can perpetuate biases present in training data. Monitoring helps find and correct biases, ensuring fair and equitable outcomes across different demographic groups.
Toxicity Monitoring: AI-driven platforms may produce or amplify toxic content, like harmful language or offensive behavior. Observability helps track and mitigate toxic outputs to maintain safe and respectful interactions.
Privacy & Security: AI systems can expose sensitive data or be vulnerable to attacks. Observability safeguards against breaches, ensuring compliance with privacy standards.
Model Drift Monitoring: AI models can become less accurate over time as the data they were trained on diverges from real-world scenarios. Observability detects this "model drift" and enables timely model updates to maintain relevance and accuracy.
How AI Observability Diagnoses Issues Faster
AI observability detects and diagnoses performance issues within AI systems much more comprehensively. Here is how:
Real-Time Detection
Real-time detection boosts the speed and efficiency of AI systems by enabling teams to identify and tackle issues the moment they arise. Organizations can continuously monitor subtle changes or unusual behaviors, allowing engineers to intervene before minor problems escalate into major ones. This proactive approach is especially important in complex AI environments, where small errors can quickly snowball into major failures.
Real-time detection also lets teams take immediate corrective actions—whether by updating the model, adjusting inputs, or rolling back to a stable version. This swift response minimizes downtime and prevents costly disruptions, which is crucial in high-stakes areas such as financial trading, healthcare, and consumer services. Rapid detection and response can prevent significant financial losses, protect patient health, and avoid poor user experiences in these contexts.
Identifying Hallucinations Faster
AI observability tools offer features like output validation, anomaly detection, and confidence scoring. Output validation and anomaly detection work collectively to cross-reference responses with trusted knowledge bases and flag deviations from expected patterns.
Confidence scoring then assigns certainty levels to responses, helping detect when a model might be hallucinating. These features, combined with a knowledge graph, make it easier for organizations to detect hallucinations in AI outputs.
Watch Now: Why Should AI Developers Care about AI Hallucinations
Automation and Continuous Feedback for Optimal AI Performance
Automation further strengthens real-time detection, as advanced algorithms monitor AI models around the clock. These automated systems detect issues faster than human operators and often suggest corrective measures, which reduces the time it takes to fix problems and minimizes the risk of human error.
Observability tools provide detailed insights through visual dashboards, allowing teams to pinpoint exactly where a problem lies. This efficient diagnosis and response process creates a continuous feedback loop, where teams monitor AI models and constantly improve them in response to new data or changing conditions. Ultimately, this approach ensures AI systems maintain optimal performance and adapt quickly and effectively to any new challenges.
Privacy and Security Monitoring
AI observability greatly improves privacy and security monitoring by providing tools that identify and address vulnerabilities. Two important features in this framework are the Detect Prompt Injection Validator and the Secrets Present Validator.
The Detect Prompt Injection Validator monitors attempts to manipulate Large Language Models (LLMs) with harmful prompts. It ensures that only safe inputs get processed by the model.
Meanwhile, the Secrets Present Validator scans outputs to ensure sensitive information, like API keys or passwords, isn’t accidentally exposed. If it finds such information, it replaces it with asterisks to protect it. These tools work together to maintain the security and integrity of AI systems.
Beyond security, AI observability helps detect problems like prompt injections or secret exposures, reducing the response time needed. Similarly, automated threat classification cuts down on the need for manual reviews. Lastly, comprehensive logging and reporting tools provide useful insights that enable faster diagnosis and informed decision-making.
Bias and Fairness Monitoring
AI observability enables continuous fairness monitoring by tracking metrics like demographic parity and equal opportunity to assess bias in real time. To facilitate this, relevant demographic attributes must be fed into metric calculators that compute fairness metrics.
Furthermore, segmented performance analysis enables organizations to identify uneven model behavior across various subgroups, such as age groups or geographic regions.
The observability system enhances this segmented analysis by tagging data points with metadata that indicates subgroup membership. This allows for easy querying and comparing performance metrics across different segments, such as accuracy, precision, and recall. Automated comparative analysis simplifies the task by generating periodic reports that stakeholders can review to identify any disparities in model behavior.
In addition, adaptive thresholds and alerts allow organizations to respond promptly to potential issues. These thresholds adjust dynamically based on historical trends. When fairness metrics exceed predefined limits, alerting systems notify stakeholders, enabling timely intervention.
Enhanced Toxicity and Content Monitoring
AI models can sometimes generate harmful or offensive content, especially when malicious users attempt to manipulate the system with toxic language. AI observability uses real-time content filtering to combat this. It automatically scans outputs for inappropriate language and harmful concepts. This proactive approach catches and addresses potentially damaging content before it reaches users.
AI observability tools can help organizations assess the emotional tone of AI-generated content by integrating sentiment analysis models. If the AI crosses predefined thresholds for negative sentiment, the system automatically alerts stakeholders, enabling immediate action.
Continuous monitoring adds another layer of protection by tracking emotional shifts over time. The system visualizes these changes using time-series analysis and detects anomalies to flag unusual spikes in negative sentiment, signaling potential issues that need attention.
AI observability, powered by knowledge graphs, enhances toxic content monitoring by providing a deeper understanding of language and context. Knowledge graphs capture the semantic relationships between words and phrases. This enables AI models to interpret nuanced language, including slang and evolving toxic expressions that might otherwise go unnoticed.
Knowledge graphs enrich word embeddings with graph-based context, clarifying whether a term has benign, harmful, or both meanings. This improved understanding enables the system to detect toxic content, even when offensive language is subtle or implied.
Managing Data Drift
Data drift occurs when the statistical properties of input data change over time, negatively impacting model performance. This shift may occur due to changes in user behavior, market conditions, sensor calibration, or other factors that cause the production data to differ from the training data.
AI observability tackles this challenge by continuously monitoring input data, using drift detection algorithms, and offering real-time visualization dashboards to quickly spot anomalies. Automated retraining mechanisms kick in when significant data drift is detected, ensuring models stay relevant and effective.
Additionally, feature importance monitoring helps track the relationships between features and outcomes. This ensures stable performance and helps organizations maintain consistency.
Knowledge graphs further enhance the management of data drift by mapping relationships between data entities and their attributes. These structured representations help identify shifts in key relationships over time. AI observability tools can identify these shifts and provide early warnings when critical relationships change. They also allow models to dynamically integrate new information, making them more adaptable to changes and reducing the impact of drift on performance.
Managing Model Degradation
Over time, models can experience a drop in performance due to factors like data drift, concept drift, environmental changes, or even adversarial attacks. This decline, known as model degradation, reduces a model's predictive accuracy and effectiveness. AI observability addresses this issue by continuously tracking key performance metrics such as accuracy, precision, recall, F1-score, and loss functions on fresh data.
Analyzing erroneous predictions in real-time helps identify patterns contributing to performance dips. Organizations can also benchmark against industry standards to ensure the model stays on track. AI observability can trigger alerts whenever performance drops are detected.
Pythia AI: Building Reliable AI Through Observability
Pythia AI offers a powerful observability platform that addresses the challenges of AI model degradation head-on. It provides continuous monitoring, real-time alerting, and advanced tools to quickly detect and resolve performance issues.
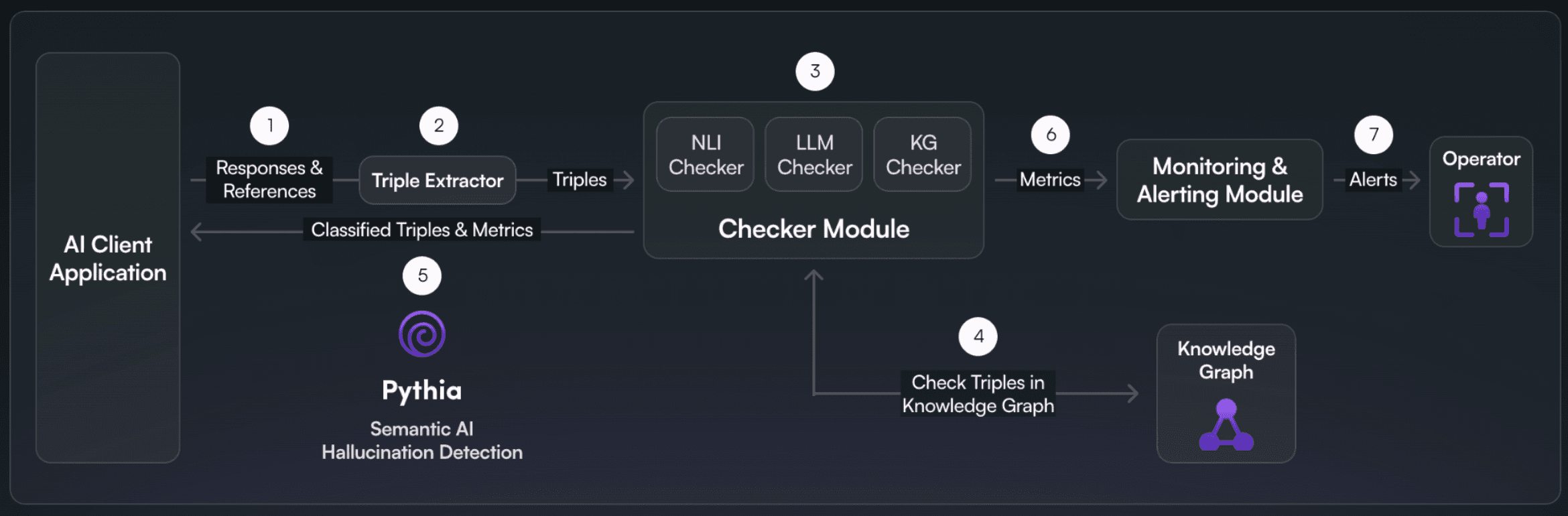
Tackling the Black Box Problem
As mentioned above, traditional AI models often function like "black boxes," making it hard to understand how they work or make decisions. This lack of transparency creates several problems, including difficulty identifying errors, unnoticed biases, etc.
Pythia AI overcomes these limitations by offering continuous observability. The platform closely monitors AI models through real-time monitoring, allowing organizations to quickly spot and address deviations from expected behavior. Pythia provides greater insights into model behavior, empowering teams to use them more effectively.
The Triplet-Based Approach
A key innovation in Pythia’s platform is its triplet-based approach, which breaks down information into knowledge triplets: subject, predicate, and object. For example, in the sentence "Marie Curie discovered radium," the triplet would be (Marie Curie, discovered, radium). This structure allows Pythia to analyze data more deeply and understand relationships between entities.
This approach brings several advantages. First, it enables a more in-depth analysis by helping the system grasp the full context of a situation rather than relying solely on keyword matching. Second, it improves the accuracy of the model’s outputs, ensuring the information generated is both correct and contextually appropriate.
Lastly, Pythia’s triplet-based method excels at detecting AI hallucinations. Therefore, it can flag inaccuracies in real-time by comparing triplets with known facts.
Categorizing AI Claims for Better Monitoring
Pythia also categorizes AI-generated claims into four types: entailment, contradiction, neutral claims, and missing facts.
Entailment refers to claims that align with both the AI output and reference data.
Contradictions highlight errors where the AI output conflicts with or lacks reference data.
Neutral claims are unverified by reference data but may still be valid.
Missing facts point to relevant information that the AI output failed to include.
Categorizing claims helps organizations gain detailed insights into the types of errors or omissions in AI responses. It improves reliability by allowing models to be refined, reduces contradictions, and helps stakeholders prioritize issues based on the severity of errors.
Measuring Accuracy
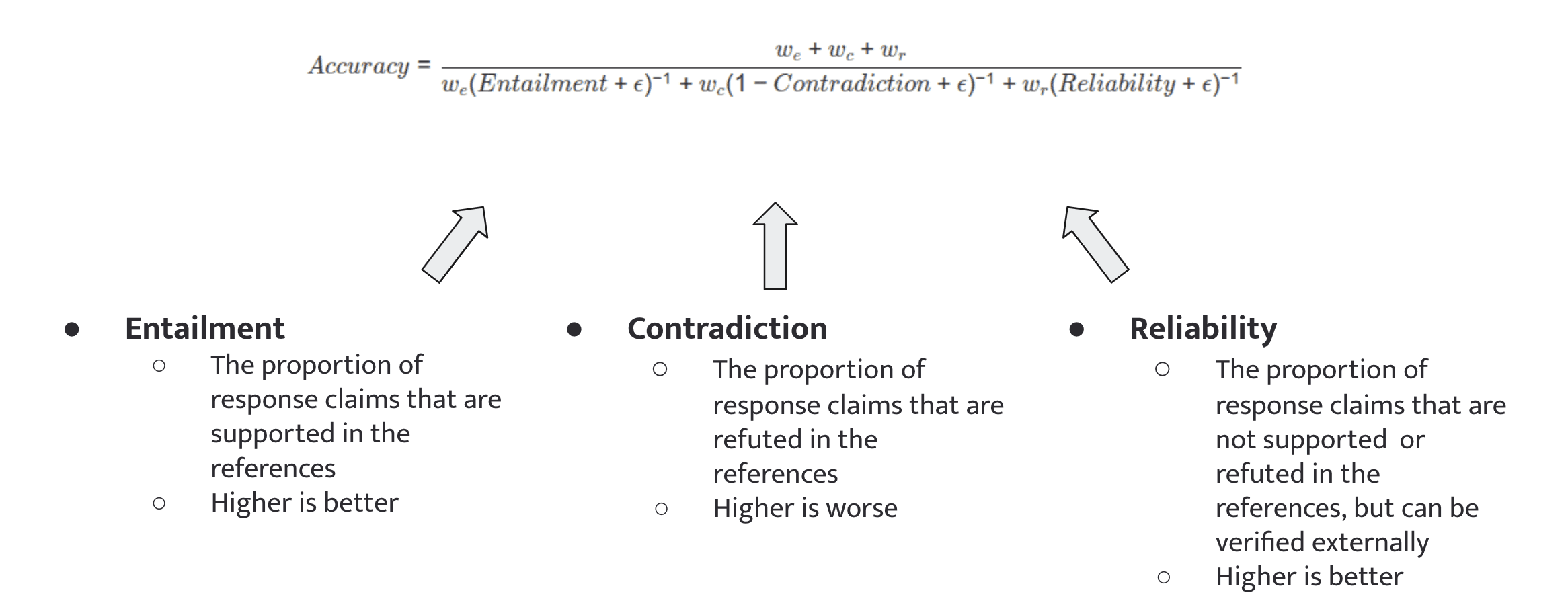
Pythia’s accuracy measurement analyzes the frequency of entailment, contradiction, and reliability in AI outputs. Entailment measures how much of the AI’s output matches verified data, with high entailment signaling reliable information. Contradiction measures conflicting claims, alerting organizations to potential errors. Pythia’s reliability metric combines both to give a holistic view of model performance. Therefore, organizations can easily identify areas that need improvement.
Learn More: How Pythia Enabled 98.8% LLM Accuracy for a Pharma Company
Conclusion
AI observability is transforming how organizations monitor and manage their AI models. While generative AI presents unprecedented opportunities for innovation and efficiency, it also brings new challenges, such as model drift, hallucinations, and biases. Tools like Pythia AI’s observability platform address these challenges head-on, ensuring that AI systems remain reliable, transparent, and effective.
Pythia’s observability platform continuously tracks and analyzes the factors that impact AI model performance. Features like hallucination detection and integration with knowledge graphs provide a deeper understanding of model behavior, allowing teams to maintain AI models that are accurate, trustworthy, and adaptable.
If you're ready to boost the reliability of your AI models, contact us today to learn how Pythia AI can help your organization achieve excellence in AI observability.